As we have seen in other articles, machine learning in medical diagnostics is transforming the way healthcare professionals detect and treat diseases. This innovative technology makes it possible to analyze large amounts of medical data with an accuracy and speed that surpasses human capabilities. In this article, we will explore how machine learning is being used to diagnose various diseases and the algorithms that are used to make these diagnoses.
What is Machine Learning in Medical Diagnostics?
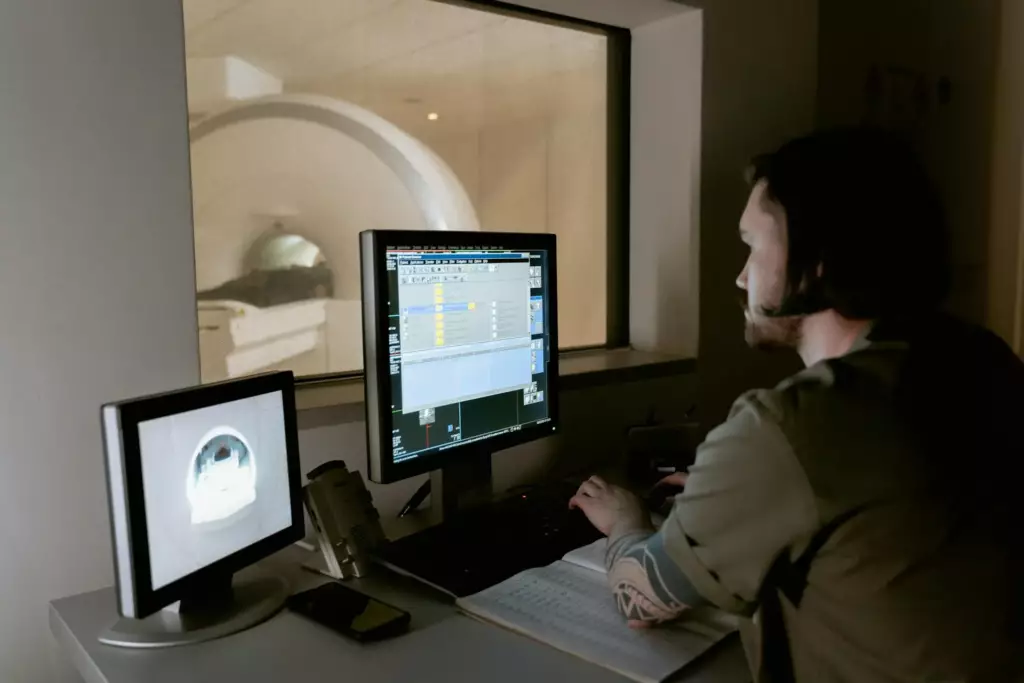
Machine learning is a branch of artificial intelligence that focuses on developing algorithms that can learn and make predictions based on data. In medical diagnostics, machine learning is used to analyze patient data, such as medical images, electronic health records and laboratory test results, to identify patterns and predict the presence of disease.
Of course in order to increase the likelihood that the medical diagnosis will be accurate, the AI review should be corroborated by a medical specialist. We can see AI as another specialist in the diagnostic team that helps to identify abnormalities more accurately.
Diseases Diagnosed with Machine Learning
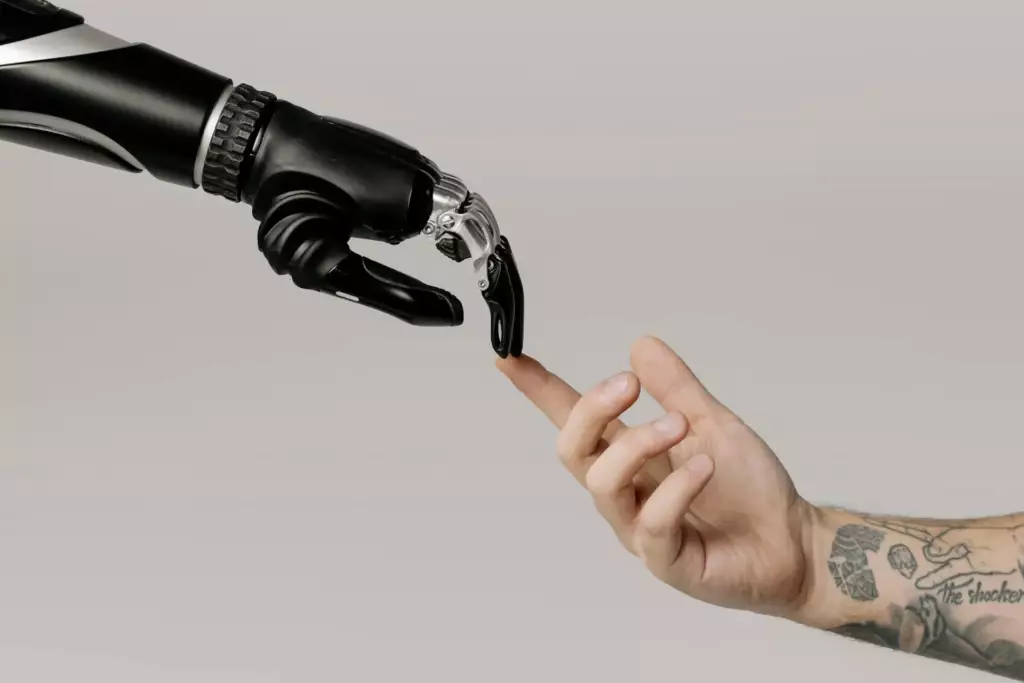
1. Cancer
Machine learning in medical diagnostics has been shown to be especially effective in the early detection of several types of cancer, including:
- Breast cancer:machine learning algorithms analyze mammograms to identify tumors and microcalcifications with high accuracy.
- Skin cancer: Machine learning tools can differentiate between benign and malignant lesions in dermatological images.
- Lung cancer: also machine learning models analyze CT scans to detect lung nodules that may be cancerous.
2. Cardiovascular diseases
Machine learning in medical diagnostics also plays a crucial role in the detection and management of cardiovascular diseases:
- Myocardial infarction: Algorithms can predict the probability of a heart attack based on electrocardiogram (ECG) data and other clinical indicators.
- Heart failure: in the same way, predictive models help identify patients at risk of developing heart failure by analyzing their medical history and test results.
3. Neurological Diseases
Neurological diseases are another field where machine learning in medical diagnostics is making significant advances:
- Alzheimer’s: machine learning algorithms analyze magnetic resonance imaging (MRI) and cognitive test data to detect early signs of Alzheimer’s.
- Epilepsy: in addition, machine learning models can predict seizures by analyzing electroencephalogram (EEG) data.
3. Infectious Diseases
During the COVID-19 pandemic, machine learning in medical diagnostics has been crucial for disease management:
- COVID-19: Algorithms analyze X-ray images and CT scans of the chest to detect COVID-19 infections. They have also been used to predict disease progression and response to treatment.
Algorithms Used in Machine Learning in Medical Diagnostics
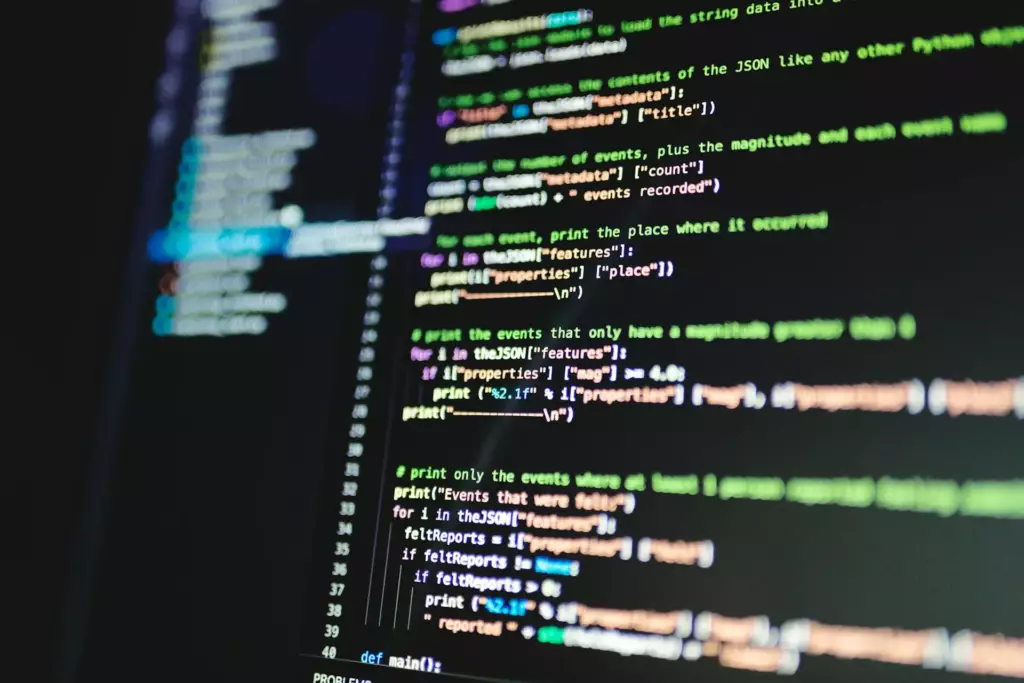
Several machine learning algorithms are used in medical diagnostics, each suitable for different types of data and problems:
1. Convolutional Neural Networks (CNN)
CNNs are particularly effective for medical image analysis. They are used in the detection of cancer, eye diseases and other conditions that can be identified by high-resolution images.
- Example: Breast cancer detection from mammograms.
2. Support Vector Machines (SVM)
Also, SVMs are useful for classifying complex data and can be applied in the analysis of text, image and signal data.
- Example: Classification of normal and abnormal heart rhythms.
3. Decision Trees and Random Forests
These algorithms are easy to interpret and very effective in prediction based on structured data sets.
- Example: Prediction of diabetes based on factors such as age, weight, medical history and other indicators.
4. Recurrent Neural Networks (RNN)
RNNs are ideal for analyzing sequential and temporal data, such as ECG and EEG signals.
- Example: Prediction of seizures in patients with epilepsy.
5. Regression Models
Regression models, such as logistic regression, are used to predict the probability of discrete events, such as the presence or absence of a disease.
- Example: Predicting the probability of myocardial infarction based on risk factors.
Conclusion
In conclusion, machine learning in medical diagnostics is revolutionizing the way diseases are detected and treated. From early detection of cancer to predicting cardiovascular and neurological diseases, machine learning algorithms are improving the accuracy and efficiency of medical diagnosis. With the continuous advancement of technology and the accumulation of health data, the future of machine learning in medical diagnostics promises to be even more promising, offering new hope for the improvement of global health. We are undoubtedly facing a great revolution for humanity, as AI is now a tool from which we can all benefit, and as we saw in this article, medicine is a clear example.