Today, recommendation algorithms have revolutionized the way we interact with online content. Starting from streaming platforms to online stores, these algorithms are designed to personalize our experience by suggesting content that we are likely to like. Throughout this article, we will explore how recommendation algorithms work, where they are used and their impact on our decisions as users.
What are Recommendation Algorithms?
Recommendation algorithms are systems that analyze data to suggest products, services or content to users. They use various data analysis and machine learning techniques to predict user preferences and make personalized recommendations.
How Recommendation Algorithms Work
Recommendation algorithms can be classified into several types according to the technique they use:
- Collaborative Filtering: This approach is based on the preferences and behaviors of a large number of users. There are two main subtypes:
* User-Based Collaborative Filtering: Recommends products that similar users have preferred.
* Item-based Collaborative Filtering: Recommends products similar to those that the user has preferred in the past.
- Content-Based Filtering: This method analyzes the characteristics of products that a user has preferred and recommends items with similar characteristics. Additionally, it uses product descriptions, metadata and other relevant information to find matches.
- Hybrid Algorithms: Combine multiple techniques, such as collaborative and content-based filtering, to improve the accuracy of recommendations. Netflix, for example, uses a hybrid approach to suggest movies and series.
- Deep Learning-Based Models: Finally, these use deep neural networks to analyze large volumes of data and capture complex patterns in user preferences. As a result, these models are highly effective in personalizing recommendations.
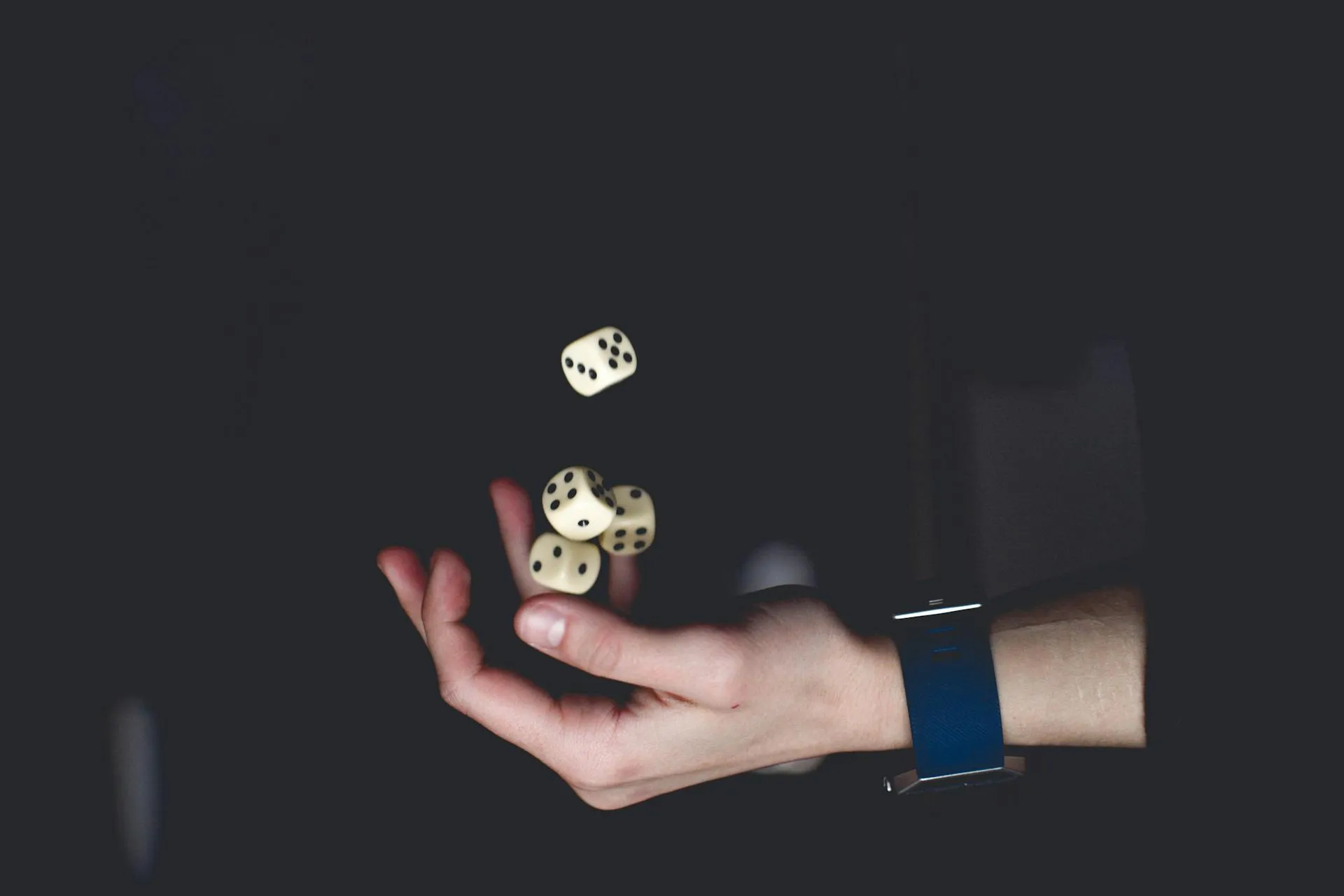
Where Recommendation Algorithms Are Used
Recommendation algorithms are present in a variety of platforms and services that we use on a daily basis. Some of the most common examples include:
1. Streaming Platforms.
Services such as Netflix, Spotify and YouTube use recommendation algorithms to suggest movies, series, music and videos based on users’ viewing history and preferences. These personalized recommendations enhance the user experience and increase the time spent interacting with the platform.
2. Online Stores.
Similarly, Amazon, eBay and other e-commerce platforms rely on recommendation algorithms to suggest products to shoppers. By analyzing past shopping behavior and searches, these stores can offer relevant product recommendations, often resulting in increased sales.
3. Social Networking
In the same way, Facebook, Instagram and Twitter use recommendation algorithms to personalize content in users’ newsfeed. By analyzing previous interactions, such as likes, comments and shares, these platforms can display content that users are more likely to be interested in.
4. News services.
Also Platforms like Google News and Flipboard use recommendation algorithms to suggest news articles based on previous reads and user preferences. This helps users discover news and topics of interest to them more efficiently.
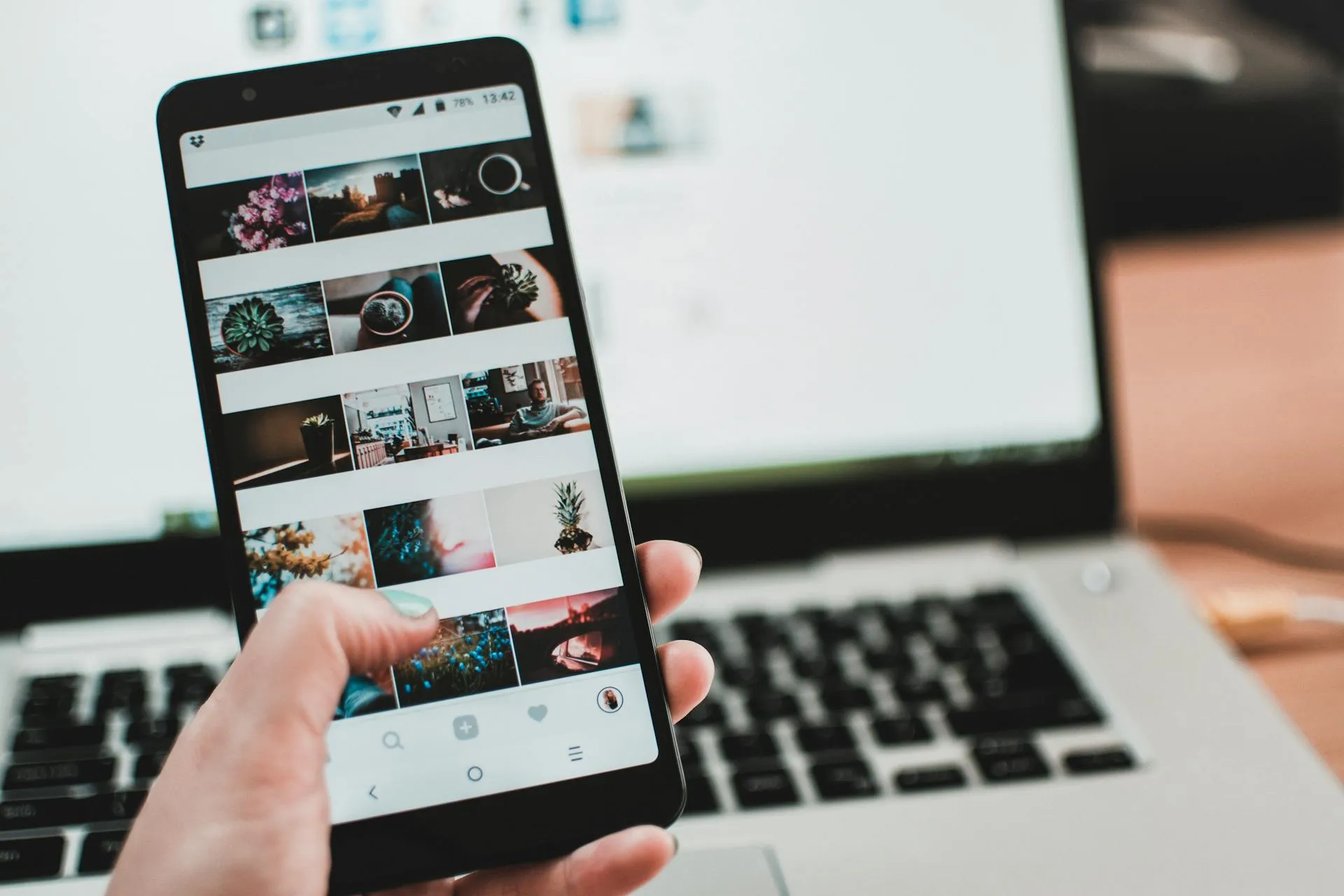
Impact of Recommendation Algorithms on Users’ Decisions
The use of recommendation algorithms has a significant impact on our decisions as users, both positively and negatively.
Advantages
- Personalization: Recommendation algorithms enhance the user experience by personalizing content and suggesting products they are likely to like.
- Time Saving: Similarly, by filtering relevant content, these algorithms help users find what they are looking for faster, saving time and effort.
- Discovery of New Content: Users can discover new movies, music, products and items they might not otherwise have found.
Challenges and Concerns
- Filter Bubble: Recommendation algorithms can limit users’ exposure to different views and diverse content, creating a “filter bubble” where only information that matches their previous preferences is displayed.
- Privacy: Also collecting and analyzing large amounts of user data raises concerns about privacy and security of personal information.
- Algorithmic Dependency: Finally, over-reliance on algorithmic recommendations can reduce the ability of users to make independent decisions and explore options outside of the algorithm’s suggestions.
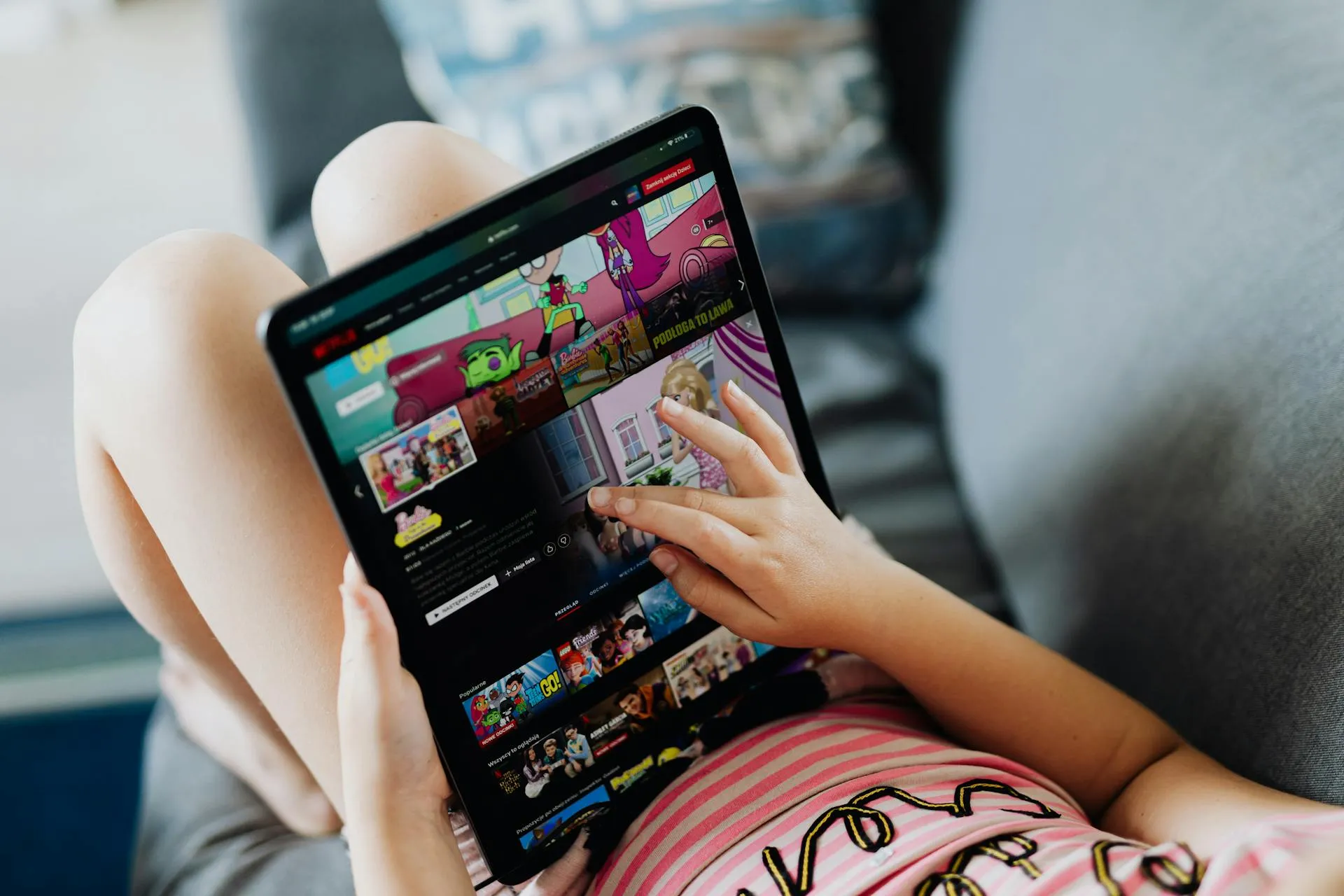
Conclusion
In conclusion, recommendation algorithms are powerful tools that have transformed the way we interact with online content. From improving personalization and new product discovery to posing challenges in terms of privacy and information diversity, these algorithms have a profound impact on our daily decisions. For this reason, as technology continues to advance, it will be crucial to address these challenges in order to take full advantage of the benefits of recommendation algorithms.